USING MATHS TO TAME CANCER COMPLEXITY
Mathematical Approaches to Modeling Metabolic Plasticity and Heterogeneity in Melanoma (Project MALMO)
Cutaneous melanoma is a highly invasive tumor and despite recent therapeutic advances, most patients with advanced melanoma have a poor clinical outcome. At the molecular level, the most frequent mutations in melanoma affect the BRAF oncogene, a protein kinase of the MAPK pathway. Therapies targeting BRAF/MEK are effective for only 50% of the patients and almost systematically generate resistance. Some non-genetic mechanisms of drug resistance are associated with the strong heterogeneity and the plasticity and melanoma cells that still remain poorly understood. In MALMO we address the importance of metabolic plasticity in melanoma cells in the context of drug resistance. In order to understand the mechanistic origin of the resistance to targeted therapies, we build a predictive multiscale mathematical model. This model describes intracellular dynamics of the metabolic pathways and the dynamics of the melanoma cell subpopulations in interaction with their micro-environment. The model has spatial extension and takes into account cellular heterogeneity. Model initial conditions and parameters describing the microenvironment are learned from image analysis of tumour sections using deep learning as segmentation approach. In order to validate the model, we use a multiplexed imaging technique applied to the detection of metabolic markers in samples prepared from murine xenografted tumours submitted to treatment. Using the mathematical model and the in situ imaging data, we study the role of the metabolic reprogramming in generating melanoma heterogeneity and its contribution to resistance to targeted therapies.
Collaborating teams: Laurent Le Cam @ IRCM, Daniel Racoceanu @ ICM, Emmanuel Faure @ LIRMM.
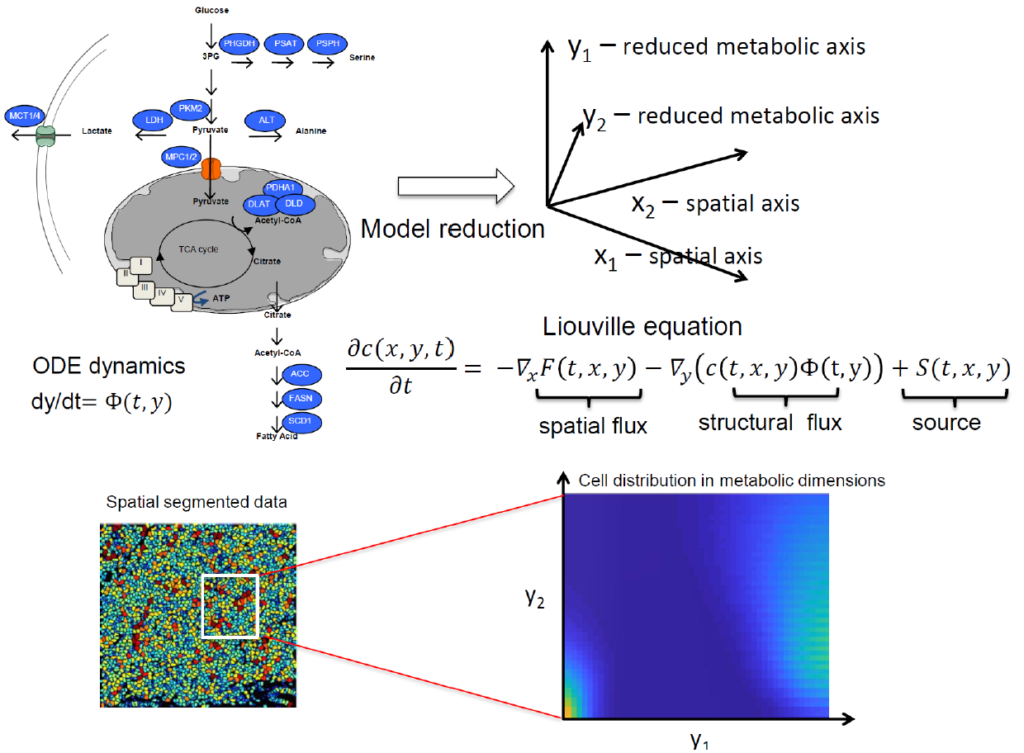
Mesoscale spatio-structural mathematical modeling of the metabolic remodeling of cancer cells. The metabolic network includes fatty acid synthesis, the de novo serine synthesis and the pyruvate pathway. Expression of the enzymes indicated in blue circles is monitored by multiplexed tissue imaging. The ODE dynamics of the metabolic network is reduced to a few dynamical variables representing metabolic dimensions. Dynamics of cell distributions in space and structure dimensions are described by the Liouville equation. The model contains other space dependent drug, extracellular matrix and chemotactic variables that are not represented in this scheme and whose dynamics is coupled to the Liouville equation. The spatial granularity of the model (white square sliding window) depends on the spatial heterogeneity of the sample: it is small enough to separate distinct cell areas, big enough to allow for cell distribution estimates.
A systems biology-based approach to tyrosine phosphorylation signaling pathways involved in breast cancer suppression (Project PhYSiCa).
The complex nature of cancer hampers traditional biological approaches to decipher its molecular mechanisms and develop targeted drug therapies. Alternative to descriptive and empirical approaches we develop a combination of biochemical and mathematical modeling of cancer mechanisms. Cancer affects a number of “hallmark” cellular processes controlled by multiple signaling pathways that interact in a complex dynamical system. Our goal is to unravel how signaling pathways affect tumor development and progression. Global (phospho)proteomic approaches are used in order to identify kinase and phosphatase substrates and interactors, but generate a large body of data that are difficult to interpret. Only “apparent” signaling pathways can be extracted from a manual scrutiny of the data. Complexity increases when taking into account their spatio-temporal regulation. In collaboration with the team of Peter Coopman at IRCM , we use systems biology methods to cope with this complexity. The architecture of the signaling networks is completed with phospho-proteomic data produced by the team of Peter Coopman or obtained from the literature and extant databases. The proteomic studies are used to select important processes and simplified network topologies that are studied using differential equations taking into account spatio-temporal activities.

References
- Buffard, A. Naldi, M. Deckert, RM. Larive O. Radulescu, PJ Coopman. The comparison of Syk signaling networks reveals the potential molecular determinants of its tumor promoter or suppressor functions. 2020, accepted Biomolecules.
- Marion Buffard, Aurélien Naldi, Ovidiu Radulescu, Peter J. Coopman, Romain M. Larive, Gilles Freiss. Network Reconstruction and Significant Pathway Extraction Using Phosphoproteomic Data from Cancer Cells. Proteomics, 2019, 19 (21-22) : 1800450.
- Arran Hodgkinson, Laurent Le Cam, Dumitru Trucu, and Ovidiu Radulescu, Spatio-Genetic and Phenotypic Modelling Elucidates Resistance and Re-Sensitisation to Treatment in Heterogeneous Melanoma, Journal of Theoretical Biology, 2019, 466:84-105.
- Satya Swarup Samal, Jeyashree Krishnan, Ali Hadizadeh Esfahani, Christoph Lüders,Andreas Weber and Ovidiu Radulescu, Metastable regimes and tipping points of biochemical networks with potential applications in precision medicine, in Automated Reasoning for Systems Biology, Pietro Lio and Paolo Zuliani etds., 2019, Springer, pp 269-295.
- A Hodgkinson, G Uzé, O Radulescu, D Trucu. Signal propagation in sensing and reciprocating cellular systems with spatial and structural heterogeneity. Bulletin of mathematical biology, (2018) 1-37.
- A.Naldi, R.M.Larive, U.Czerwinska, S.Urbach, P.Montcourrier, C.Roy, J.Solassol, G.Freiss, P.J.Coopman and O.Radulescu. Reconstruction and Signal Propagation Analysis of the Syk Signaling Network in Breast Cancer Cells. PLOS Computational Biology (2017) 13: e1005432.